Public Article
-
verified
Prediction of user throughput in the mobile network along the motorway and trunk road
ISSN: 2744 - 2527Publisher: author
Prediction of user throughput in the mobile network along the motorway and trunk road
Indexed in
Technology and Engineering
ARTICLE-FACTOR
1.3
Article Basics Score: 2
Article Transparency Score: 2
Article Operation Score: 2
Article Articles Score: 2
Article Accessibility Score: 2
SUBMIT PAPER ASK QUESTION
International Category Code (ICC):
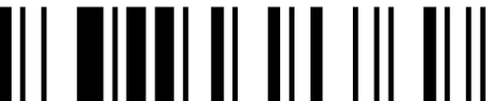
ICC-1802
Publisher: "ims" Vogosca
International Journal Address (IAA):
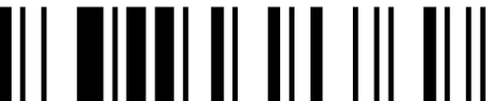
IAA.ZONE/2744395682527
eISSN
:
2744 - 2527
VALID
ISSN Validator
Abstract
The main goal of this research is to create a machine learning model for predicting user throughput in the mobile 4G network of the network provider M:tel Banja Luka, Bosnia and Herzegovina. The geographical area of the research is limited to the section of Motorway "9th January" (M9J) Banja Luka - Doboj, between the node Johovac and the town of Prnjavor (P-J section), and the area of the section of trunk road M17, between the node Johovac and the town of Doboj (J-D section). Based on the set of collected data, several models based on machine learning techniques were trained and tested together with the application of the Correlation-based Feature Selection (CFS) method to reduce the space of input variables. The test results showed that the models based on k-Nearest Neighbors (k-NN) have the lowest relative prediction error, for both sections, while the model created for the trunk road section has significantly better performance.
Basics |
Contact and Support |
For authors |
Legal |
Home Evaluation | Contact Us
Facebook Twitter |
Guide for authors
indexarticle
|
Terms & Conditions |